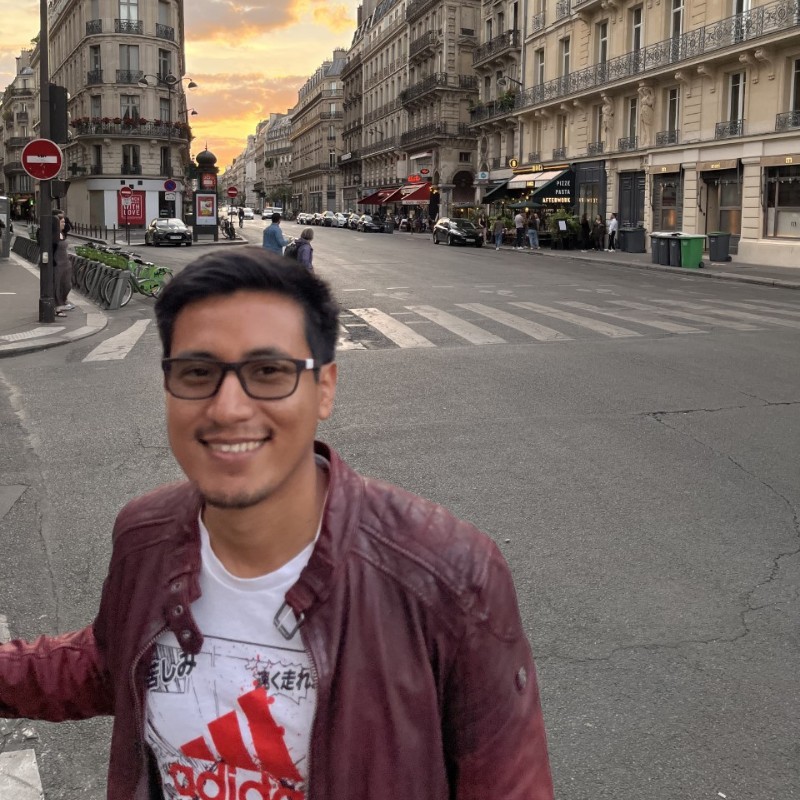
Student: Carlos Escudero Castillo
MS in Data Science (Concentration in Computational Data Analytics)
MS in Data Science (Concentration in Computational Data Analytics)
MS in Data Science (Concentration in Computational Data Analytics)
Ph.D., Computer Science, University of Wisconsin-Madison
Ph.D., Electrical and Computer Engineering, University of Illinois at Chicago (UIC)
Postdoctoral Fellow at Florida International University
Epilepsy is a neurological disorder of the brain that causes seizures, which can be defined as short changes in the normal brain activity that can cause a variety of temporary changes in both perception and behavior. Epileptic seizures are signs of epilepsy, the second-most severe dynamic condition of the brain after strokes and these cannot be managed by any anti-convulsant drugs in today’s times.
Seizure prediction is a field that holds great promise for elucidating the dynamical mechanisms underlying the disorder and enabling implantable devices to act in time to treat epilepsy. Seizure prediction technology has been the focus of more than 30 years of international research with the goal of relieving the burden of unpredictability and integrating fresh, time-specific treatment. Clinical trials to evaluate prospective prediction and intervention methodology and devices for Food and Drug Administration (FDA) approval are now being conducted in academic institutions and the medical business due to the explosion of interest in this field that have led to the emergence of new EEG datasets, seizure prediction contests, and further prospective study that aim to improvements and better comprehension of the mechanisms behind seizures.
Through this thesis, we contend that all these developments, when combined with data analyses, pave the way for renewed work on seizure prediction approaches. To encourage the creation of a remedy that eases the burden of the unpredictable nature of seizures, this thesis suggests new lines of inquiry incorporating a synergy between mechanisms, models, data, devices, and algorithms and contribute to the current standards for the development of seizure prediction technology and pave the way for further research for more enthusiasts in this field.
There is currently a lack of standardization in the sampling and model evaluation protocols for machine learning-based seizure prediction. This can lead to challenges in comparing different models and may result in overly optimistic results. The duration T between two clusters is known as the seizure-free time T because seizures frequently occur in clusters. A leading seizure is the first seizure in each cluster. According to Chen et al., T should be determined by examining natural seizure clusters, and they advised selecting the natural cluster with the longest length as the value of T. To see natural seizure clusters, most EEG recordings in available databases are too brief. As a compromise between getting actual leading seizures and getting positive samples, a value of T = 4 hr which is frequently used in relevant studies is chosen.
The seizure prediction horizon (SPH) and seizure occurrence period (SOP) are indeed important parameters for sampling in seizure prediction studies. The SPH refers to the length of time that the model predicts ahead of a seizure event, while the SOP refers to the period of time during which a seizure is considered to be occurring. Different studies may use different values for these parameters, which can make it challenging to compare the results directly. A prediction alarm is considered successful if it detects a seizure within the SOP (seizure occurrence period) and with a lead time of at least the SPH (seizure prediction horizon) before the seizure onset. A prediction that occurs outside the SOP or within the SOP but with less than the required SPH lead time is considered a false positive or a missed prediction.
The choice of values for the SPH and SOP parameters in seizure prediction studies can have important implications for patient care and well-being. If the SPH is too short (like 0 sec), patients may not have enough time to prepare for protective measures before the onset of a seizure, while if the SPH is too long (in some studies 1 hr to 4 hr), there may be unnecessary stress and anxiety for patients. Similarly, if the SOP is too short, there may not be enough preictal samples to train the model effectively, while if the SOP is too long, there may be a risk of premature anxiety and unnecessary stress for patients. Therefore, it is important for researchers to carefully consider the balance between the needs for patient safety and model accuracy when selecting values for the SPH and SOP parameters. The used SPH and SOP are 5 and 30 minutes, respectively. An interictal condition that occurs at least two hours before or after a seizure is taken into account to make sure the segment being studied is sufficiently removed from the preictal interval as shown in above figure. We create a sample set for the model's training to divide these segments into smaller chunks using the moving window method.